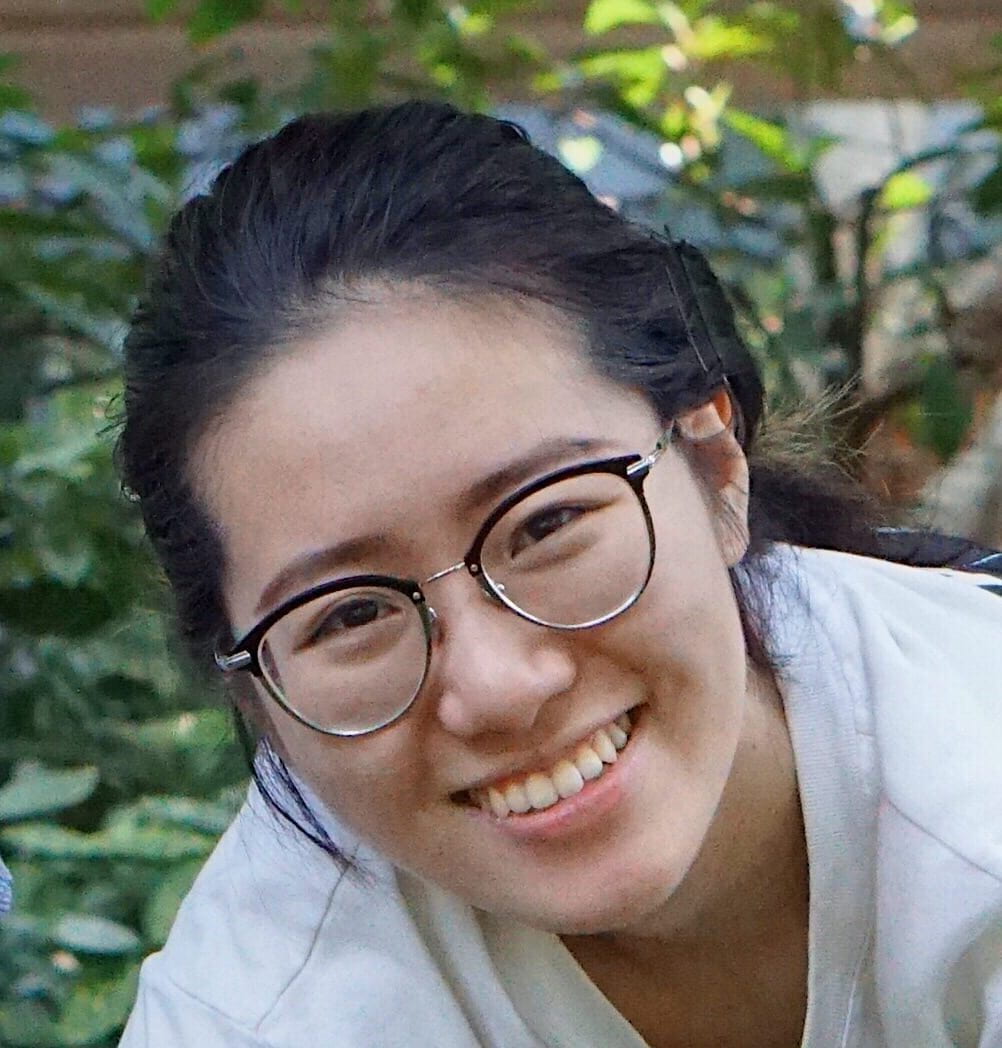
NeurIPS 2021 Lead Author Spotlight
Ran Liu, PhD Machine Learning student
Drop, Swap, and Generate: A Self-Supervised Approach for Generating Neural Activity
#COMPUTATIONAL NEUROSCIENCE
We introduced a novel unsupervised approach for learning disentangled representations of neural activity called SwapVAE. Our approach combines a generative modeling framework with an instance-specific alignment loss that tries to maximize the representational similarity between transformed views of the input (brain state). Through evaluations on both synthetic data and neural recordings from hundreds of neurons in different primate brains, we show that it is possible to build representations that disentangle neural datasets along relevant latent dimensions linked to behavior.

Q&A with Ran Liu
(click question to show answer)
What motivated your work on this paper?
Brain activities are often complex and noisy, yet it is believed by neuroscientists that a low-dimensional neural representation that governs neural signals exists. The biggest motivation of this project is to find a low-dimensional representation space that could ‘explain’ the neural signals. Our work SwapVAE presents an initial step towards this goal by combining self-supervised learning (SSL) techniques with the generative modeling framework to learn interpretable representations of neural activities.
If readers remember one takeaway from the paper, what should it be and why?
It should be our latent space augmentation operation BlockSwap. BlockSwap makes the latent representation more interpretable by separating the latent representation into augmentation-invariant information and augmentation-variant information, and swapping the invariant part before reconstruction. We hope BlockSwap can be applied in other scenarios when interpretability matters.
Were there any “aha” moments or lessons that you’ll use to inform your future work?
My lesson was summarized 2000 years ago by Aristotle: “For the things we have to learn before we can do them, we learn by doing them.”
What are you most excited for at NeurIPS and what do you hope to take away from the experience?
I am excited to check out other cutting-edge works! I hope to learn from the computational neuroscientists to see how they approach similar problems, and am also excited to learn from other deep learning scientists for inspiration.
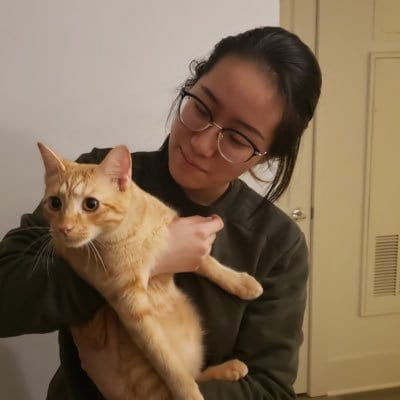

NeurIPS 2021 Lead Author Spotlight
Guan-Horng Liu, PhD Machine Learning student
Second-Order Neural ODE Optimizer
#SECOND-ORDER OPTIMIZATION
We propose a novel second-order optimization framework for training the emerging deep continuous-time models, specifically the Neural Ordinary Differential Equations (Neural ODEs). Since their training already involves expensive gradient computation by solving a backward ODE, deriving efficient second-order methods becomes highly nontrivial. Nevertheless, inspired by the recent Optimal Control (OC) interpretation of training deep networks, we show that a specific continuous-time OC methodology, called Differential Programming, can be adopted to derive backward ODEs for higher-order derivatives at the same O(1) memory cost. Our code is available at https://github.com/ghliu/snopt.
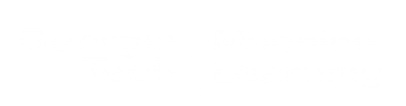
Q&A with Guan-Horng Liu
(click question to show answer)
What motivated your work on this paper?
Despite that the adjoint-based optimization process of Neural ODEs is originated from Optimal Control Principle, few attempts have been made along this principle. We are interested in seeing the practical improvements it can bring to the table.
If readers remember one takeaway from the paper, what should it be and why?
That the deep learning optimization is fundamentally intertwined with optimal control principle paves an elegant path towards principled training improvement and new applications that will otherwise remain unexplored in the current framework.
Were there any “aha” moments or lessons that you’ll use to inform your future work?
Math won’t lie 🙂
What are you most excited for at NeurIPS and what do you hope to take away from the experience?
I am definitely excited to learn more at NeurIPS.

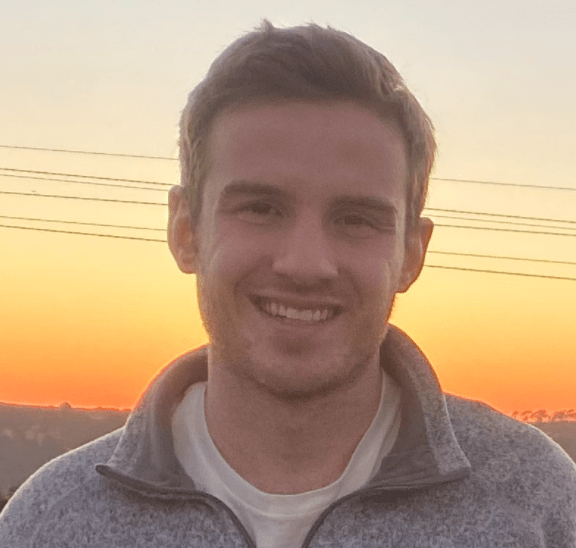
NeurIPS 2021 Lead Author Spotlight
Andrew Szot, PhD Machine Learning student
Habitat 2.0: Training Home Assistants to Rearrange their Habitat
#MACHINE LEARNING
We introduce Habitat 2.0 (H2.0), a simulation platform for training virtual robots in interactive 3D environments and complex physics-enabled scenarios. We make comprehensive contributions to all levels of the embodied AI stack – data, simulation, and benchmark tasks. Specifically, we present: (i) ReplicaCAD: an artist-authored, annotated, reconfigurable 3D dataset of apartments (matching real spaces) with articulated objects (e.g. cabinets and drawers that can open/close); (ii) H2.0: a high-performance physics-enabled 3D simulator with speeds exceeding 25,000 simulation steps per second (850x real-time) on an 8-GPU node, representing 100x speed-ups over prior work; and, (iii) Home Assistant Benchmark (HAB): a suite of common tasks for assistive robots (tidy the house, stock groceries, set the table) that test a range of mobile manipulation capabilities. These large-scale engineering contributions allow us to systematically compare deep reinforcement learning (RL) at scale and classical sense-plan-act (SPA) pipelines in long-horizon structured tasks, with an emphasis on generalization to new objects, receptacles, and layouts. We find that (1) flat RL policies struggle on HAB compared to hierarchical ones; (2) a hierarchy with independent skills suffers from ‘hand-off problems’, and (3) SPA pipelines are more brittle than RL policies.
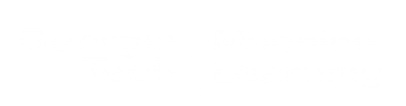
Q&A with Andrew Szot
(click question to show answer)
What motivated your work on this paper?
I was motivated to work on this paper to accelerate embodied AI towards being able to complete realistic tasks in the home such as cooking meals, loading the dishwasher, or cleaning up. Previously, working towards this goal was challenging due to the lack of datasets, slow simulators, and missing benchmarks to measure progress. Motivated by these three deficiencies, our work proposed a dataset of interactive house-scale scenes, a simulator that is 100x faster than prior work, and a benchmark for realistic tasks in the home.
If readers remember one takeaway from the paper, what should it be and why?
One takeaway is that Habitat 2.0 is the perfect test bed for developing agents in interactive, 3D, and physics-enabled tasks in the home. Also that researchers should get started using the dataset, simulator, and benchmark at https://aihabitat.org.
Were there any “aha” moments or lessons that you’ll use to inform your future work?
A part of the project was benchmarking how prior approaches performed on long and complex tasks in the home such as preparing groceries or setting the table. An “aha” moment was realizing that all previous methods achieved zero success on the hardest version of the benchmark. This finding informs my future research in learning algorithms that address such long and compositional task structures.
What are you most excited for at NeurIPS and what do you hope to take away from the experience?
I am excited to meet other researchers in the space. I hope to take away new connections from the experience.

NeurIPS 2021 Lead Author Spotlight
Hassan Mortagy, PhD Operations Research student
Reusing Combinatorial Structure: Faster Iterative Projections over Submodular Base Polytopes
#CONVEX OPTIMIZATION
Optimization algorithms such as projected Newton’s method, FISTA, mirror descent and its variants enjoy near-optimal regret bounds and convergence rates, but suffer from a computational bottleneck of computing “projections” in potentially each iteration (e.g., O(T1/2) regret of online mirror descent). On the other hand, conditional gradient variants solve a linear optimization in each iteration, but result in suboptimal rates (e.g., O(T3/4) regret of online Frank-Wolfe). Motivated by this trade-off in runtime v/s convergence rates, we consider iterative projections of close-by points over widely-prevalent submodular base polytopes B(f). We develop a toolkit to speed up the computation of projections using both discrete and continuous perspectives. We subsequently adapt the away-step Frank-Wolfe algorithm to use this information and enable early termination. For the special case of cardinality based submodular polytopes, we improve the runtime of computing certain Bregman projections by a factor of Ω(n/log(n)). Our theoretical results show orders of magnitude reduction in runtime in preliminary computational experiments.

Q&A with Hassan Mortagy
(click question to show answer)
What motivated your work on this paper?
In practice, we always encounter the tradeoff between the performance of an algorithm and its runtime. It is often the case that algorithms that have optimal theoretical performance suffer from computational bottlenecks that cause their running times to be very high and restrictive in practice. However, in some settings having optimal performance is desirable and necessary since that would translate to better revenue for example. The questions is, should we give up on those algorithms despite their optimal performance? In this paper, we make an argument that the answer to that question is no. In particular, we attempt to eliminate computational bottlenecks within some optimal machine learning and optimization algorithms (such as mirror descent), with the aim of improving their runtimes by orders of magnitude to make these algorithms competitive in practice, while maintaining their optimal performance.
If readers remember one takeaway from the paper, what should it be and why?
In this work, we bridge discrete and continuous optimization insights to eliminate projections bottlenecks appearing within optimal optimization algorithms such as mirror descent.
Were there any “aha” moments or lessons that you’ll use to inform your future work?
This has to be to always keep the big picture and practical impact of your work in mind. Also, that submitting to NeurIPS is a goal that should always be in your mind while doing the research and not after the research is done; this allows you to adapt the work to the “NeurIPS way” as you go along.
What are you most excited for at NeurIPS and what do you hope to take away from the experience?
I am really excited to see the latest cutting-edge research, and hope to network and make as many connections as I can.


NeurIPS 2021 Lead Author Spotlight
Alejandro Carderera, PhD Machine Learning student
Simple steps are all you need: Frank-Wolfe and generalized self-concordant functions
#OPTIMIZATION
We prove that a simple step size achieves a O(1/t) convergence rate in primal gap and in dual gap for a simple variant of the Frank-Wolfe algorithm when minimizing generalized self-concordant functions over compact convex domains, using only first-order information. Previous approaches achieved a O(1/t) convergence rate in primal gap using first and second-order information.

Q&A with Alejandro Carderera
(click question to show answer)
What motivated your work on this paper?
We wanted to come up with a simple 5-line optimization algorithm that would achieve the same convergence guarantees as more complex algorithms in the literature for minimizing generalized self-concordant functions in the projection-free case.
If readers remember one takeaway from the paper, what should it be and why?
The main takeaway is that simple algorithms are usually more robust than more complex algorithms, and in some special cases they can achieve similar convergence properties!
Were there any “aha” moments or lessons that you’ll use to inform your future work?
When we were scouting the literature we realized that a lot of the algorithms for minimizing this class of functions were complex, they utilized expensive operations, and they achieved sublinear convergence. We realized that with a few simple tricks, and with much cheaper operations we could theoretically prove the same properties, while being computationally cheaper to run.
What are you most excited for at NeurIPS and what do you hope to take away from the experience?
I’m excited to meet other researchers working on similar topics, and I’m sure that new and interesting ideas will spring from these interactions at the conference.


NeurIPS 2021 Lead Author Spotlight
Sheng Zhang, PhD Machine Learning student
Finite Sample Analysis of Average-Reward TD Learning and Q-Learning
#REINFORCEMENT LEARNING
Our work establishes the first finite-sample convergence guarantees in the literature of average-reward reinforcement learning algorithms: (i) average-reward TD learning with linear function approximation for policy evaluation and (ii) average-reward tabular Q-learning to find an optimal policy. Analysis of average-reward reinforcement learning algorithms is known to be more challenging to study than their discounted-reward counterparts. The key property that is exploited in the study of discounted-reward problems is the contraction property of the underlying Bellman operator. In the average-reward setting, such a contraction property does not hold under any norm, and the Bellman equation is known to have multiple fixed points. To resolve this difficulty, we construct Lyapunov functions using projection and infimal convolution to analyze the convergence of equivalent classes generated by these algorithms. Our approach is simple and general, so we expect it to have broader applications in other problems.

Q&A with Sheng Zhang
(click question to show answer)
What motivated your work on this paper?
Recent literature obtains finite sample guarantees for discounted-reward TD learning and Q-learning algorithms. Such a study of average-reward RL algorithms is not undertaken, which are known to be more challenging to study than their discounted-reward counterparts.
If readers remember one takeaway from the paper, what should it be and why?
One takeaway: We establish the first finite sample convergence guarantees of average-reward TD learning with linear function approximation and average-reward tabular Q-learning in the literature.
Reason: The theoretical understanding of average-reward RL methods is quite limited.
Were there any “aha” moments or lessons that you’ll use to inform your future work?
We realized that our approach is simple and general, so we expect it to have broader applications in other problems.
What are you most excited for at NeurIPS and what do you hope to take away from the experience?
It is a great opportunity to learn the state-of-the-art research on various machine learning problems. I hope to find some research ideas for my future work.

NeurIPS 2021 Lead Author Spotlight
Rohan Paleja, PhD Robotics student
The Utility of Explainable AI in Ad Hoc Human-Machine Teaming
#EXPLAINABLE AI
We present two human-subject studies quantifying the benefits of deploying Explainable AI (xAI) techniques within a human-machine teaming scenario, finding that the benefits of xAI are not universal. We create a rich, interactive human-machine teaming scenario in Minecraft where a human and collaborative robot (i.e., a cobot) must work together to build a house. We show that xAI techniques providing an abstraction of the cobot’s behavior can support situational awareness (SA) and examine how different SA levels induced via a collaborative AI policy abstraction affect ad hoc human-machine teaming performance. Our work presents one of the first analyses looking at the impact of explainable AI in collaborative sequential decision-making settings. Our results demonstrate that researchers must deliberately design and deploy the right xAI techniques in the right scenario by carefully considering human-machine team composition and how the xAI method augments SA.

Q&A with Rohan Paleja
(click question to show answer)
What motivated your work on this paper?
Given my prior work in interpretable machine learning, I was interested in identifying the utility of explainable AI (xAI) approaches when deployed to complex, human-machine teaming domains. Furthermore, I was hoping that deploying several xAI approaches within a human-machine teaming setting would reveal key drawbacks in the real-world practicability of current xAI approaches and inspire my future work in developing xAI for high-performance human-machine teaming.
If readers remember one takeaway from the paper, what should it be and why?
Full explainability, providing complete information about a collaborative robot’s policy, is preferred by users prior to task execution. However during task execution, partial explainability, which provides a low-level abstraction of the collaborative robot’s policy, proves more beneficial.
We hope this takeaway can inform other researchers in their design of xAI approaches, modifying the design appropriately based on whether the approach is online (during task execution) or offline (before or after task execution).
Were there any “aha” moments or lessons that you’ll use to inform your future work?
When running human-subjects studies, assess/plot your data often. You may detect new and thought-provoking patterns that can further inform your experimental analysis and lead to interesting conclusions.
What are you most excited for at NeurIPS and what do you hope to take away from the experience?
I’m excited to present my research and hope to both meet other researchers in the field and discover interesting future directions.
